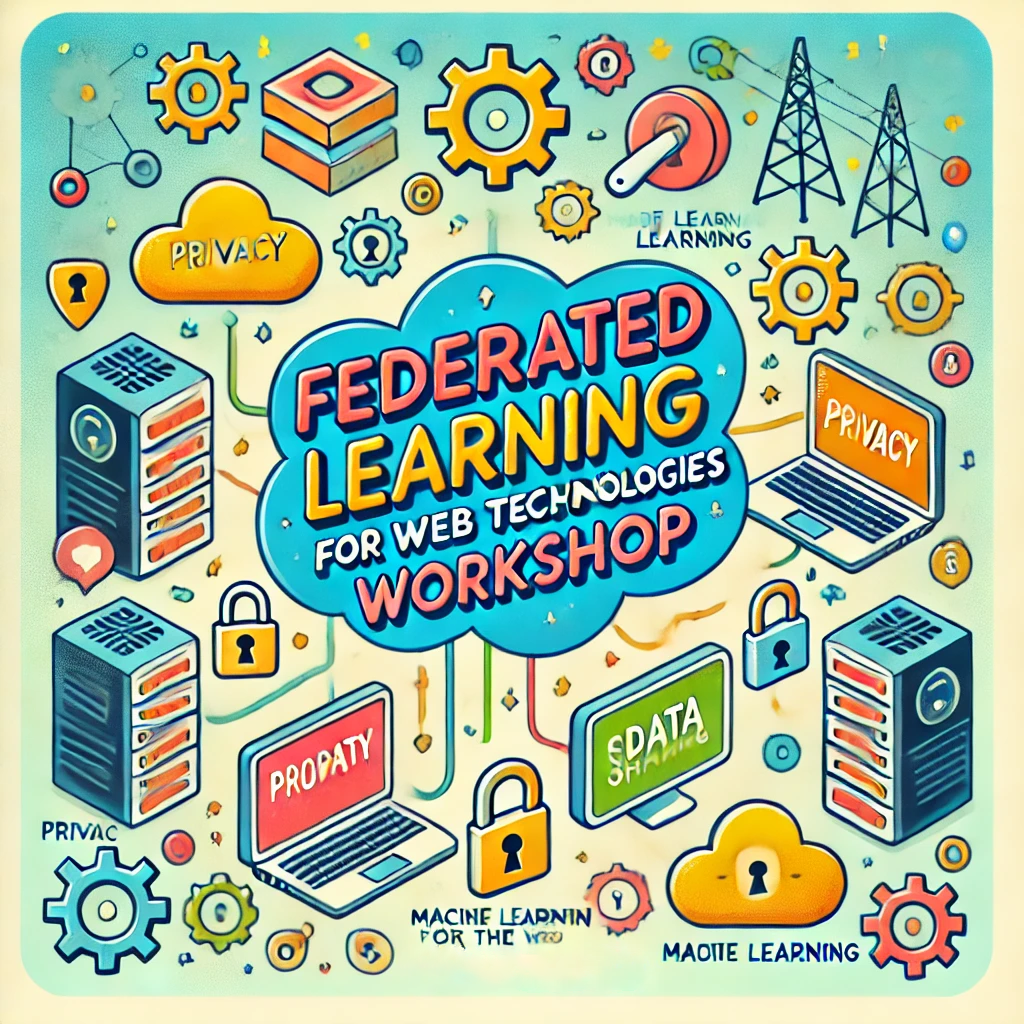
Workshop FL4WEB @ICDCS 2025
FL4WEB – Federated Learning for Web Technologies
Organizers
- Prof. Francesco Piccialli, University of Naples Federico II
- Dr. Fabio Giampaolo, University of Naples Federico II
- Dr. Diletta Chiaro, University of Naples Federico II
- Dr. Mirko Polato , University of Turin, Italy
- Prof. Yang Liu, Tsinghua University, China
List of Accepted Papers
ID | Titolo | Autori |
---|---|---|
57 | An adaptive weighted federated learning algorithm based on ShapDiv | Tian Zhu Jia and Yu Hong Zhao |
69 | MSPSL: An Efficient Multi-Server Parallel Split Learning | Xiaofeng Lu, Yinhui Li and Pietro Lio |
78 | FPML: Heterogenous Federated Learning based on Deep Mutual Learning | Yong Li, Hao Chen, Han Gao, Yiming Wang and Yongxin Jiang |
94 | A Federated Learning Framework for Wind Power Forecasting | Faiza Loukil, Youssef El-Kouch, Hervé Verjus and Flavien Vernier |
105 | Improving Class-Level Fairness Under Non-IID Data Distributions in Federated Learning | Sarhad Arisdakessian, Omar Abdel Wahab, Osama Wehbi, Azzam Mourad and Hadi Otrok |
109 | A Adversarial Approach for Urban Anomaly Detection Across Completion Spatiotemporal Data | Xinghong Jiang, Yong Ma, Changhao Jin, Jiang Luo, Yunni Xia and Yongzhao Zhang |
112 | FedBGS: A Blockchain Approach to Segment Gossip Learning in Decentralized Systems | Fabio Turazza, Marcello Pietri, Marco Picone and Marco Mamei |
116 | Benchmarking Federated Learning Under Realistic Non-IID Conditions: A Structured Partitioning Approach Using ImageNet | Tatjana Legler, Vinit Hegiste and Martin Ruskowski |
117 | Trade-Off Between Communication Rounds and Local Epochs in Federated Learning | Vinit Hegiste, Tatjana Legler and Martin Ruskowski |
118 | Partial Federated Primal-Dual Optimization for Network-Based Android Malware Detection | Mohammad Mashreghi and Mohammad Hossein Badiei |
119 | AgentFL: AI-Orchestrated Agents for Federated Learning | Viraaji Mothukuri and Reza Parizi |
Primary Contact
Email: francesc.piccialli@unina.it
Abstract
Artificial Intelligence (AI)-based systems, especially those based on machine learning technologies, have become central in modern societies. In the meanwhile, users and legislators are becoming aware of privacy issues. Users are increasingly reluctant in sharing their sensitive information, and new laws have been enacted to regulate how private data is handled (e.g., the GDPR). Federated Learning (FL) has been proposed to develop better AI systems without compromising users’ privacy and the legitimate interests of private companies. Although FL is still an emerging technology, it has already shown significant theoretical and practical results making FL one of the hottest topics in the machine learning community. Given the considerable potential in overcoming the challenges of protecting users’ privacy while making the most of available data, we propose a workshop on Federated Learning for the Web Technologies (FL4WEB) at the 45th IEEE International Conference on Distributed Computing Systems (IEEE ICDCS 2025). The goal of this workshop is to focus the attention of the ICDCS research community on addressing the open questions and challenges in this thriving research area. Given the broad range of competencies in the ICDCS community, the workshop will welcome foundational contributions as well as contributions expanding the scope of these techniques, such as improvements in the interpretability and fairness of the learned models.
Important Dates
Submission Deadline: March 10 (EXTENDED), 2025
Author Notification: April 03, 2025
Camera-ready Submission: April 16, 2025
Scope and Topics of Interest
- Algorithmic and theoretical advances in FL for the Web
- Federated Learning for personalization (e.g., FL in recommender systems and user modeling)
- Security and privacy of FL systems (e.g., differential privacy, adversarial attacks, poisoning attacks, inference attacks, data anonymization, model distillation, secure multi-party computation
- Other non-functional properties of FL for the Web (e.g., fairness, interpretability/explainability, personalization
- Federated Learning with heterogeneous data/model distributions
- FL variants and Decentralized Federated Learning (e.g., vertical FL, split learning, gossip learning
- Applications/Use cases of FL for the Web
- Demos, tools and resources (e.g., benchmark datasets, software libraries)
Submission System and Important Details
The workshop paper submission system will be the EasyChair ICDCSW 2025: Easychair Submission Link
Submissions to the Workshop must be original and unpublished and must not be submitted concurrently for publication elsewhere. All submissions should follow the IEEE 8.5″ x 11″ two-column format using 10pt fonts and the IEEE Conference template (downloadable by selecting “Conferences” in the IEEE-Template Selector https://template-selector.ieee.org/). Each submission can have up to 11 pages (including figures, tables, appendices, and references). Submissions exceeding this page limit or with smaller fonts will be desk-rejected without review. The review process is singe/double-blind review. For each accepted paper, at least one author is required to register and attend the workshop in-person to present their poster/paper on-site. No-show paper will be reported to the publisher and removed from the ICDCSW companion conference proceedings. All accepted and presented papers will be included in the IEEE ICDCSW companion conference proceedings and IEEE digital library.
Note that the authors should adhere to ethic and professional standards of IEEE. Please refer to IEEE Code of Ethics and IEEE Policy of AI-Generated Text. >
Program Committee
To be Completed
- Antonella Guzzo, University of Calabria, Italy
- Jerry Chun-Wei Lin, Silesian University of Technology, Poland
- Pian Qi, University of Naples Federico II, Italy
- Edoardo Prezioso, University of Naples Federico II, Italy
- Stefano Izzo, University of Naples Federico II, Italy
- Daniela Annunziata, University of Naples Federico II, Italy
- Martina Savoia, University of Naples Federico II, Italy
- David Camacho, Universidad Politecnida de Madrid, Spain