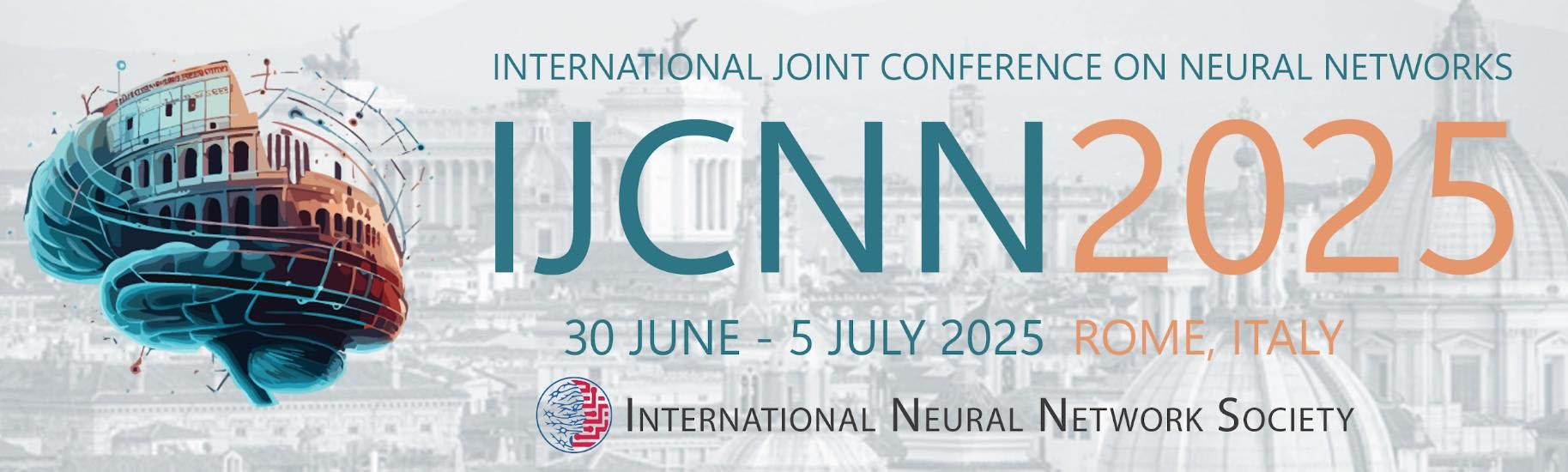
Special Session: RobustFL
Towards Robust Federated Learning: Addressing Data and Device Heterogeneity
Organizers
- Dr. Diletta Chiaro, University of Naples Federico II
- Prof. Francesco Piccialli, University of Naples Federico II
- Dr. Fabio Giampaolo, University of Naples Federico II
Primary Contact
Email: diletta.chiaro@unina.it
Abstract
Federated Learning (FL) has emerged as a groundbreaking approach to enabling AI across decentralized and sensitive data sources without requiring data centralization, addressing privacy concerns that align with regulations like GDPR. For a leading conference like IJCNN, which emphasizes advancements in neural networks and transformative AI, FL offers a rich avenue for exploration. While past sessions at IJCNN (see IJCNN 2024) have largely focused on privacy and trustworthiness, the next critical frontier is addressing the inherent challenges of non-iid data distributions and device heterogeneity—barriers that must be overcome for FL to scale and perform effectively in real-world settings.
In practical FL deployments, data generated across decentralized sources is not only non-iid but is also produced by diverse devices with varying computational power, network stability, and memory capacity. This device heterogeneity complicates learning, as not all devices can contribute equally or reliably. Such variability can degrade model performance, create biases, and complicate both convergence and fairness. For example, FL applications in healthcare and IoT span a range of devices, from powerful servers to lightweight edge devices, each with unique data characteristics and resource constraints. Addressing non-iid data and device heterogeneity is essential for FL to be scalable, fair, and ethically viable, especially in critical areas like medical diagnostics and financial forecasting.
Important Dates
Submission Deadline: January 15, 2025
Scope and Topics of Interest
- Algorithmic and Theoretical Advances: New algorithms that improve FL’s performance and adaptability to both non-iid data and heterogeneous device constraints.
- Fairness and Robustness: Strategies for creating models that are fair and resilient across varying data sources and device capacities.
- Interpretability and Explainability: Approaches to ensure transparency and interpretability in FL models that operate across diverse data and devices.
- Personalization and Client Adaptivity: Techniques for personalizing FL models to specific clients while maintaining overall robustness, despite differing device capabilities and data.
- Resource-Aware FL: Frameworks that optimize FL for resource-limited devices, accounting for constraints in memory, battery life, and connectivity.
- Application Case Studies: Real-world applications demonstrating FL’s performance in managing non-iid data and device heterogeneity, particularly in sensitive areas like healthcare and IoT.
- Tools and Benchmarks: Development of benchmark datasets and evaluation metrics that reflect the challenges of both non-iid data and diverse device conditions.
Program Committee
- Afaf Taïk, Mila – Quebec AI Institute
- Afsana Khan, Maastricht University
- Alessio Mora, University of Bologna
- Alessio Masano, Università degli studi di Catania
- Bingjie Yan, Institute of Computing Technology, Chinese Academy of Sciences
- Bostan Khan, Mälardalens University
- Chaochao Sun, Shanghai University of Electric Power
- Daniela Annunziata, University of Naples Federico II
- Danilo Menegatti, Sapienza University of Rome
- Depeng Chen, Anhui University
- Diletta Chiaro, Università degli Studi di Napoli Federico II
- Divya Kulkarni, Harvard Medical School – Massachusetts General Hospital
- Enrique Tomás Martínez Beltrán, University of Murcia
- Fabio Giampaolo, University of Naples Federico II
- Giovanni Paragliola, CNR
- Guanglei Yu, 新疆医科大学
- Hanyue Xu, School of AI and Advanced Computing, Xi’an Jiaotong-Liverpool University
- Haokun Chen, University of Munich
- Jayant Vyas, Indian Institute of Technology Jodhpur
- Jiayi Mao, Zhejiang University
- Jing Xu, School of Computer and Communication Engineering, Changsha University of Science and Technology
- Judith Sáinz-Pardo Díaz, Spanish National Research Council (CSIC)
- Jue Xiao, Huazhong University of Science and Technology
- Junfeng Chen, Jinan University
- Kejia Zhang, School of Computer Science and Big Data (School of Cybersecurity) Heilongjiang University
- Ljubomir Rokvic, EPFL Lorenzo Valerio, IIT-CNR
- Manasa Mariam Mammen, Mercedes-Benz AG
- Manuel Röder, Technical University of Applied Sciences Würzburg-Schweinfurt
- Marco Garofalo, University of Pisa
- Mario Colosi, University of Messina
- Martina Savoia, University of Naples Federico II
- Marzia Canzaniello, University of Naples Federico II
- Melike Geçer, University of Lausanne
- Mengchen Fan, University of the Alabama at Birmingham
- Mingjia Shi, Sichuan University
- Mingkai Hu, Henan University of Science and Technology
- Monika Farsang, Technische Universität Wien (TU Wien)
- Mrinmay Sen, Indian Institute of Technology Hyderabad
- Nan Yang, The University of Sydney
- Neena Goveas, BITS Pilani, Goa
- Obaidullah Zaland, Umeå University
- Oudom Kem, CEA
- Paul Zheng, RWTH Aachen University
- Pian Qi, University of Naples Federico II
- Raman Zatsarenko, Rochester Institute of Technology
- Renyou Xie, Central South University
- Samuele Fonio, Università degli studi di Torino
- Sheng Wan, The Hong Kong University of Science and Technology
- Sileshi Nibret Zeleke, University of Bari, Department of Computer Science
- Songlian Yan, 华南师范大学
- Tianxiang Chen, Beihang University
- Truong Thao Nguyen, National Institute of Advanced Industrial Science and Technology (AIST)
- Wai Fong Tam, Queen Mary University of London
- Xuwei Fan, Zhejiang Gongshang University
- Yan Zhou, Kunsan National University
- Yansong Zhao, Nanyang Technological University
- Yichen Li, Huazhong University of Science and Technology
- Yihang Wu, 桂林电子科技大学
- Yiming Chen, Tsinghua University
- Yitao Chen, Arizona State University
- Youngjoon Lee, KAIST
- Yu Huo, The Chinese University of Hong Kong, Shenzhen
- Zahra Batool, Mila – Quebec AI Institute
- Zhe Li, Rochester Institute of Technology